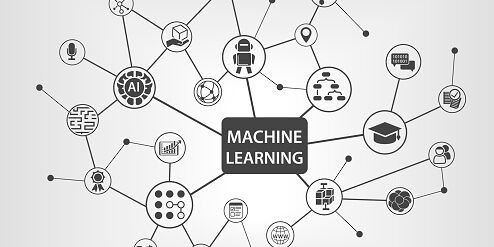
In the fast-paced realm of technology, the evolution of machine learning has revolutionized industries, from healthcare to finance, by offering groundbreaking solutions to complex problems. As this transformative technology continues to advance, its influence on the hiring landscape cannot be underestimated. In this article, we delve into the evolution of machine learning and its implications for the hiring process, highlighting the shift from an academic-centric approach to a more diverse and practical hiring landscape.
The Evolution of Machine Learning
Machine learning, a subset of artificial intelligence, has evolved significantly since its inception. Initially, machine learning algorithms relied on structured data and explicit programming to perform tasks. However, with the advent of deep learning and neural networks, machines gained the ability to learn from unstructured data, such as images and text, leading to remarkable advancements in image recognition, natural language processing, and more.
The Rise of the Academic-Centric Approach
Historically, machine learning engineering roles were often filled by individuals with strong academic backgrounds, including PhDs in fields such as computer science, mathematics, and statistics. This academic-centric approach ensured a foundation in complex algorithms and theoretical concepts, but it also has led to a potential gap between academic knowledge and real-world applications.
The Shifting Paradigm in Hiring
As machine learning technologies become more accessible and businesses recognize their potential, the hiring landscape for machine learning engineers is undergoing a paradigm shift. While academic expertise remains valuable, companies are now seeking candidates who can bridge the gap between theoretical knowledge and practical implementation. This shift is driven by the need to address real-world challenges, collaborate across multidisciplinary teams, and deliver tangible results.
Emphasis on Practical Experience
To adapt to this changing landscape, aspiring machine learning engineers are encouraged to gain practical experience alongside their academic pursuits. Participating in industry projects, internships, or open-source contributions can provide hands-on exposure to real-world problems and enhance a candidate’s ability to translate theoretical concepts into practical solutions.
Diverse Skill Set
The evolution of machine learning has expanded the skill set required for success in the field. In addition to algorithmic expertise, employers are increasingly seeking candidates with skills in data preprocessing, feature engineering, model deployment, and continuous learning. Communication and collaboration skills are also gaining prominence as engineers are expected to work across teams to integrate machine learning solutions into various business processes.
Holistic Approach to Hiring
Recognizing that machine learning is not a standalone discipline, forward-thinking companies are adopting a holistic approach to hiring. This involves building diverse teams that bring together professionals with various backgrounds, such as domain experts, software developers, and user experience designers. This multidisciplinary approach ensures a well-rounded perspective and fosters innovation by combining different skill sets.